
Not All Machine Learning Leads to Artificial Intelligence
The majority of machine learning applications aren’t quite in the realm of AI, and that isn’t likely to change anytime soon.
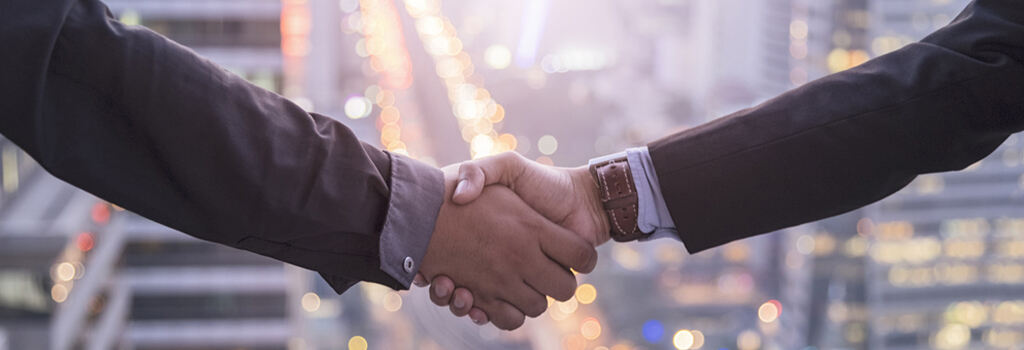
So far in this series we have seen how AI and machine learning are two different entities: AI as a product for non-expert users and machine learning as a set of tools to create products, as well as touching on how machine learning works.
To sharpen our understanding of the differences between machine learning and AI, we now want to make clear that not all machine learning activities have to result in AI. In reality, the majority of machine learning is performed for purposes other than AI.
The engine and the car…
Let’s look back at our analogy of the engine and the car, where machine learning acts as the engine to AI’s car. Now, engines can be used for many other purposes than cars. For example, one needs an engine in a motorcycle, boat or airplane. Engines are also used in power plants and various industrial production processes, as well as in home appliances such as a vacuum cleaner, grinder, electric razor, dish washer, etc. Note: none of these examples qualify as a car.
Thus, there is no direct path from an engine to a car. This is because for something to be a car, it must provide the functionality of transportation of goods and persons. By contrast, an engine provides physical force. These are two distinct functions.
Because machine learning provides a separate function to AI, it can be used for purposes other than AI. In business and academia, machine learning is commonly used to detect patterns in data; investigate the properties of signals; and many other similar purposes that do not result in an easy-to-use AI product. Rather, the result still requires significant mathematical, scientific or technical expertise.
Examples of machine learning efforts that do not qualify as AI include analyses of medical images, analyses of sequences of nucleotides in genome, investigations into how neurons in the brain communicate, or discoveries of extrasolar planets. In hands of experts, machine learning can be very useful, even without AI.
Machine learning in business
In business settings, data scientists who perform machine learning do not create an AI in most cases. Instead, they may create a ‘bare bones’ predictive model without any additional bells and whistles. In these instances, the machine does not make an automatic decision; an educated, trained expert makes the final call. The model is used as a decision-making aid.
Other models made by data scientists cannot live long without data scientists having to ‘service’ them. Their performance needs to be checked regularly, and they may need to be retrained with up-to-date data fairly frequently.
In predictive maintenance, models are developed to calculate whether a machine is likely to break in the near future. When a predictive model detects such a possibility, it informs the operators of the machine. Importantly, the final decision on whether to take pre-emptive action and initiate a pre-failure repair rests with human controllers. Humans take into account additional factors such as availability of service personnel, availability of parts, cost that would be incurred on the production process, other priorities that may have emerged, etc. At the end, the intelligence that drives maintenance decisions is human, and informed, but not dictated by machines. For that reason, the machine learning model does not qualify as an AI.
It would be different if the machine would make all the decisions autonomously, with humans acting solely to physically execute repairs. We would then call it an AI: the systems of sensors, knowledge base and software would be so advanced that humans could rely on the machine to always make the optimal decision.
There are also other applications of machine learning that (typically) do not deserve the label of AI, while still providing much value to businesses. These include image classifications, recommendation engines, and churn modelling. In each of these cases, human expertise is involved during the setup of the models, model servicing and decision making. It is through the combination of software, hardware and humans that the necessary function is performed.
The majority of machine learning applications aren’t quite in the realm of AI, and that isn’t likely to change anytime soon. This means that machine learning will continue to be used in ways that do not create an AI. AI is only one of the ways to use machine learning.
Stay tuned for the next post where we will learn about AI that does not need any machine learning.
Discover more about AI for the enterprise: https://www.teradata.com/Insights/Artificial-Intelligence.
Restez au courant
Abonnez-vous au blog de Teradata pour recevoir des informations hebdomadaires